class: left,middle,title-slide count: false # .orange[HaRAM]: .orange[**Ha**]miltonian .orange[**R**]epelling .orange[**A**]ttracting .orange[**M**]etropolis<br> ## ### .bolder[Siddharth Vishwanath & Hyungsuk Tak] --- class: center, middle count:false <iframe src="https://sidvishwanath.com/mcmc-demo/" width="90%" height="90%"></iframe> .footnote.tiny.pull-left[ </br> <br> </br> </br> Courtesy the [template by Chi Feng](https://github.com/chi-feng/mcmc-demo) ] --- class: inverse, center, middle, animated slideInUp slideOutRight count: false class: left # .left[Sampling] <div style="display:none"> $$ \newcommand{\defeq}{\stackrel{\small\bullet}{=}} \newcommand{\ra}{\rangle} \newcommand{\la}{\langle} \newcommand{\norm}[1]{\left\|#1\right\|} \newcommand{\abs}[1]{\left\lvert#1\right\rvert} \newcommand{\Abs}[1]{\Bigl\lvert#1\Bigr\rvert} \newcommand{\pr}{{\mathbb P}} \newcommand{\qr}{{\mathbb Q}} \newcommand{\muv}{{\boldsymbol{mu}}} \newcommand{\xv}{{\boldsymbol{x}}} \newcommand{\av}{{\boldsymbol{a}}} \newcommand{\bv}{{\boldsymbol{b}}} \newcommand{\cv}{{\boldsymbol{c}}} \newcommand{\dv}{{\boldsymbol{d}}} \newcommand{\ev}{{\boldsymbol{e}}} \newcommand{\fv}{{\boldsymbol{f}}} \newcommand{\gv}{{\boldsymbol{g}}} \newcommand{\hv}{{\boldsymbol{h}}} \newcommand{\nv}{{\boldsymbol{n}}} \newcommand{\sv}{{\boldsymbol{s}}} \newcommand{\tv}{{\boldsymbol{t}}} \newcommand{\uv}{{\boldsymbol{u}}} \newcommand{\vv}{{\boldsymbol{v}}} \newcommand{\wv}{{\boldsymbol{w}}} \newcommand{\zerov}{{\mathbf{0}}} \newcommand{\onev}{{\boldsymbol{1}}} \newcommand{\phiv}{{\boldsymbol{\phi}}} \newcommand{\cc}{{\check{C}}} \newcommand{\xv}{{\boldsymbol{x}}} \newcommand{\Xv}{{\boldsymbol{X}\!}} \newcommand{\yv}{{\boldsymbol{y}}} \newcommand{\Yv}{{\boldsymbol{Y}}} \newcommand{\zv}{{\boldsymbol{z}}} \newcommand{\Zv}{{\boldsymbol{Z}}} \newcommand{\Iv}{{\boldsymbol{I}}} \newcommand{\Jv}{{\boldsymbol{J}}} \newcommand{\Cv}{{\boldsymbol{C}}} \newcommand{\Ev}{{\boldsymbol{E}}} \newcommand{\Fv}{{\boldsymbol{F}}} \newcommand{\Gv}{{\boldsymbol{G}}} \newcommand{\Hv}{{\boldsymbol{H}}} \newcommand{\alphav}{{\boldsymbol{\alpha}}} \newcommand{\epsilonv}{{\boldsymbol{\epsilon}}} \newcommand{\betav}{{\boldsymbol{\beta}}} \newcommand{\deltav}{{\boldsymbol{\delta}}} \newcommand{\gammav}{{\boldsymbol{\gamma}}} \newcommand{\etav}{{\boldsymbol{\eta}}} \newcommand{\piv}{{\boldsymbol{\pi}}} \newcommand{\thetav}{{\boldsymbol{\theta}}} \newcommand{\tauv}{{\boldsymbol{\tau}}} \newcommand{\muv}{{\boldsymbol{\mu}}} \newcommand{\phiinv}{\Phi^{-1}} \newcommand{\Fiinv}{F^{-1}} \newcommand{\giinv}{g^{-1}} \newcommand{\fhat}{\hat{f}} \newcommand{\ghat}{\hat{g}} \newcommand{\ftheta}{f_\theta} \newcommand{\fthetav}{f_{\thetav}} \newcommand{\gtheta}{g_\theta} \newcommand{\gthetav}{g_{\thetav}} \newcommand{\ztheta}{Z_\theta} \newcommand{\xtheta}{\Xv_\theta} \newcommand{\ytheta}{\Yv_\theta} \newcommand{\p}{\partial} \newcommand{\f}{\frac} \newcommand{\cf}{\cfrac} \newcommand{\e}{\epsilon} \newcommand{\indep}{\perp\kern-5pt \perp} \newcommand{\inner}[1]{\langle#1\rangle} \newcommand{\pa}[1]{\left(#1\right)} \newcommand{\pb}[1]{\left\{#1\right\}} \newcommand{\pc}[1]{\left[#1\right]} \newcommand{\pA}[1]{\Big(#1\Big)} \newcommand{\pB}[1]{\Big\{#1\Big\}} \newcommand{\pC}[1]{\Big[#1\Big]} \newcommand{\ty}[1]{\texttt{#1}} \newcommand{\borel}[1]{\mathscr{B}\pa{#1}} \newcommand{\scr}{\mathcal} \newcommand{\scrb}{\mathscr} \newcommand{\argmin}{\mathop{\text{arg}\ \!\text{min}}} \newcommand{\arginf}{\mathop{\text{arg}\ \!\text{inf}}} \newcommand{\argmax}{\mathop{\text{arg}\ \!\text{max}}} \newcommand{\argsup}{\mathop{\text{arg}\ \!\text{sup}}} \newcommand{\bigo}[1]{\mathcal{O}_{p}\!\left(#1\right)} \newcommand{\f}{\frac} \newcommand{\e}{\epsilon} \newcommand{\inv}{^{-1}} \newcommand{\phiinv}{\Phi^{-1}} \newcommand{\Fiinv}{F^{-1}} \newcommand{\giinv}{g^{-1}} \newcommand{\fhat}{\hat{f}} \newcommand{\ghat}{\hat{g}} \newcommand{\ftheta}{f_\theta} \newcommand{\fthetav}{f_{\thetav}} \newcommand{\gtheta}{g_\theta} \newcommand{\gthetav}{g_{\thetav}} \newcommand{\ztheta}{Z_\theta} \newcommand{\xtheta}{\Xv_\theta} \newcommand{\ytheta}{\Yv_\theta} \newcommand{\absdet}[1]{\abs{\det\pa{#1}}} \newcommand{\jac}[1]{\Jv_{#1}} \newcommand{\absdetjx}[1]{\abs{\det\pa{\Jv_{#1}}}} \newcommand{\absdetj}[1]{\norm{\Jv_{#1}}} \newcommand{\sint}{sin(\theta)} \newcommand{\cost}{cos(\theta)} \newcommand{\sor}[1]{S\mathcal{O}(#1)} \newcommand{\ort}[1]{\mathcal{O}(#1)} \newcommand{\A}{{\mathcal A}} \newcommand{\C}{{\mathbb C}} \newcommand{\E}{{\mathbb E}} \newcommand{\F}{{\mathcal{F}}} \newcommand{\N}{{\mathbb N}} \newcommand{\R}{{\mathbb R}} \newcommand{\Q}{{\mathbb Q}} \newcommand{\Z}{{\mathbb Z}} \newcommand{\X}{{\scr{X}}} \newcommand{\Y}{{\mathbb{Y}}} % \newcommand{\G}{{\mathcal{G}}} \newcommand{\M}{{\mathcal{M}}} \newcommand{\betaequivalent}{\beta\text{-equivalent}} \newcommand{\betaequivalence}{\beta\text{-equivalence}} \newcommand{\Mb}{{\boldsymbol{\mathsf{M}}}} \newcommand{\Br}{{\mathbf{\mathsf{Bar}}}} \newcommand{\dgm}{{\mathbf{\mathsf{Dgm}}}} \newcommand{\Db}{{\mathbf{\mathsf{D}}}} \newcommand{\Img}{{\mathbf{\mathsf{Img}}}} \newcommand{\mmd}{{\mathbf{\mathsf{MMD}}}} \newcommand{\Xn}{{\mathbb{X}_n}} \newcommand{\Yn}{{\mathbb{Y}_n}} \newcommand{\Xb}{{\mathbb{X}}} \newcommand{\Yb}{{\mathbb{Y}}} \newcommand{\s}{{{\sigma}}} \newcommand{\fnsbar}{{\bar{f}^n_\s}} \newcommand{\fns}{{f^n_\s}} \newcommand{\fs}{{f_\s}} \newcommand{\fsbar}{{\bar{f}_\s}} \newcommand{\barfn}{{{f}^n_\sigma}} \newcommand{\barfnm}{{{f}^{n+m}_\sigma}} \newcommand{\barfo}{{{f}_\sigma}} \newcommand{\fn}{{f^n_{\rho,\sigma}}} \newcommand{\fnm}{{f^{n+m}_{\rho,\sigma}}} \newcommand{\fo}{{f_{\rho,\sigma}}} \newcommand{\K}{{{K_{\sigma}}}} \newcommand{\barpn}{{\bar{p}^n_\sigma}} \newcommand{\barpo}{{\bar{p}_\sigma}} \newcommand{\pn}{{p^n_\sigma}} \newcommand{\po}{{p_\sigma}} \newcommand{\J}{{\mathcal{J}}} \newcommand{\B}{{\mathcal{B}}} \newcommand{\pt}{{\tilde{\mathbb{P}}}} \newcommand{\Winf}{{W_{\infty}}} \newcommand{\HH}{{{\scr{H}_{\sigma}}}} \newcommand{\D}{{{\scr{D}_{\sigma}}}} \newcommand{\Ts}{{T_{\sigma}}} \newcommand{\Phis}{{\Phi_{\sigma}}} \newcommand{\nus}{{\nu_{\sigma}}} \newcommand{\Qs}{{\mathcal{Q}_{\sigma}}} \newcommand{\ws}{{w_{\sigma}}} \newcommand{\vs}{{v_{\sigma}}} \newcommand{\ds}{{\delta_{\sigma}}} \newcommand{\fp}{{f_{\pr}}} \newcommand{\prs}{{\widetilde{\pr}_{\sigma}}} \newcommand{\qrs}{{\widetilde{\qr}_{\sigma}}} \newcommand{\Inner}[1]{\Bigl\langle#1\Bigr\rangle} \newcommand{\innerh}[1]{\langle#1\rangle_{\HH}} \newcommand{\Innerh}[1]{\Bigl\langle#1\Bigr\rangle_{\HH}} \newcommand{\normh}[1]{\norm{#1}_{\HH}} \newcommand{\norminf}[1]{\norm{#1}_{\infty}} \newcommand{\gdelta}{{\G_{\delta}}} \newcommand{\supgdelta}{{\sup\limits_{g\in\gdelta}\abs{\Delta_n(g)}}} \newcommand{\id}{\text{id}} \newcommand{\supp}{\text{supp}} \newcommand{\cech}{\v{C}ech} \newcommand{\Zz}{{\scr{Z}}} \newcommand{\psis}{\psi_\s} \newcommand{\phigox}{\Phis(\xv)-g} \newcommand{\phigoy}{\Phis(\yv)-g} \newcommand{\fox}{{f^{\epsilon,{\xv}}_{\rho,\sigma}}} \newcommand{\prx}{{\pr^{\epsilon}_{\xv}}} \newcommand{\pro}{{\pr_0}} \newcommand{\dotfo}{\dot{f}_{\!\!\rho,\s}} \newcommand{\phifo}{{\Phis(\yv)-\fo}} \newcommand{\phifox}{{\Phis(\xv)-\fo}} \newcommand{\kinf}{{\norm{\K}_{\infty}}} \newcommand{\half}{{{\f{1}{2}}}} \newcommand{\Jx}{\J_{\epsilon,{\xv}}} \newcommand{\dpy}{\text{differential privacy}} \newcommand{\edpy}{$\epsilon$--\text{differential privacy}} \newcommand{\eedpy}{$\epsilon$--edge \text{differential privacy}} \newcommand{\dpe}{\text{differentially private}} \newcommand{\edpe}{$\epsilon$--\text{differentially private}} \newcommand{\eedpe}{$\epsilon$--edge \text{differentially private}} \newcommand{\er}{Erdős-Rényi} \newcommand{\krein}{Kreĭn} % \newcommand{\grdpg}{\mathsf{gRDPG}} % \newcommand{\rdpg}{\mathsf{RDPG}} % \newcommand{\eflip}{{\textsf{edgeFlip}}} \newcommand{\grdpg}{\text{gRDPG}} \newcommand{\rdpg}{\text{RDPG}} \newcommand{\eflip}{{\text{edgeFlip}}} \newcommand{\I}{{\mathbb I}} \renewcommand{\pa}[1]{\left(#1\right)} \renewcommand{\pb}[1]{\left\{#1\right\}} \renewcommand{\pc}[1]{\left[#1\right]} % % % \newcommand{\N}{\mathcal{N}} \newcommand{\D}{\boldsymbol{\nabla}} \newcommand{\d}[1]{{\partial}_{#1}} \newcommand{\dd}[1]{\f{d}{d #1}} % \newcommand{\ddd}[2]{\f{\partial #2}{\partial #1}} \newcommand{\ddd}[2]{\f{d #2}{d #1}} \newcommand{\q}{\boldsymbol{q}} \newcommand{\q}{\boldsymbol{q}} \renewcommand{\tr}{^{\top}} \newcommand{\e}{\epsilon} \newcommand{\qo}{{\boldsymbol{q}_0}} \newcommand{\p}{{\boldsymbol{p}}} \newcommand{\tp}{{\widetilde{\boldsymbol{p}}}} \newcommand{\z}{{\boldsymbol{z}}} \newcommand{\tz}{\widetilde{\boldsymbol{z}}} \newcommand{\init}{\textup{init}} \newcommand{\half}{{\f12}} \newcommand{\vhalf}{{1/2}} \newcommand{\F}{{\boldsymbol{\Psi}}} \newcommand{\P}{{\boldsymbol{\Phi}}} \newcommand{\flip}{\mathbf{F}} \newcommand{\vec}[1]{\begin{bmatrix}#1\end{bmatrix}} \newcommand{\S}{\Sigma} \newcommand{\Ov}{\mathbf{O}} \newcommand{\Om}{\boldsymbol{\Omega}} \newcommand{\G}{\boldsymbol{\Gamma}} % \newcommand{\inv}[1]{{}^{#1}} $$ </div> Generate samples `\(\pb{ \q_1, \q_2, \dots, \q_n } \sim \pi\)` from a target distribution .content-box-blue[ $$ \pi(\q) \propto e^{- U(\q) } $$ ] -- ### .orange[Methods] 1. .large[Rejection sampling ❌ ]<br> 2. .large[Random-walk Metropolis ]✅<br> -- 3. .large[Metropolis adjusted Langevin algorithm ]😮<br> 4. .large[Hamiltonian Monte Carlo ]😲<br> -- 5. .large[Stein Variational gradient descent ]🤯<br> 6. .large[Wasserstein gradient flows ]🤯<br> 7. .large[Normalizing flows ]🤯<br> 8. ... --- class: left count: false # .left[Objective] <br><br><br> .Large.content-box-orange[<body> Can we design a .bolder.dblue.text-shadow[sampler] which:<br><br> 1. Can efficiently sample from .bolder.dblue.text-shadow[multi-modal distributions]?<br><br> 2. .bolder.dblue.text-shadow[Preserves all the nice properties] of existing mainstays? </body>] --- class: inverse, center, middle count: false # Background ## (⚡️ Speed) <!-- --- class: left ### .dblue[Robust TDA] .large[ 1. Robust Persistent Diagrams using Reproducing Kernels] .large[ 2. Efficient and Robust Topological Inference] ### .dblue[Statistical Inference using TDA] .large[ 3. Statistical Invariance of Betti Numbers] ### .dblue[Differential Privacy under TDA Lens] .large[ 4. The Shape of Edge Differential Privacy] ### .dblue[Geometry in Multimodal Sampling and Non-convex Optimization] .large[ 5. Repelling-Attracting Hamiltonian Monte Carlo] --> <style type="text/css"> .panelset { --panel-tab-foreground: currentColor; --panel-tab-background: unset; --panel-tab-active-foreground: #0148A4; --panel-tab-active-background: unset; --panel-tab-active-border-color: currentColor; --panel-tab-hover-foreground: currentColor; --panel-tab-hover-background: unset; --panel-tab-hover-border-color: currentColor; --panel-tab-inactive-opacity: 0.3; --panel-tabs-border-bottom: #ddd; } </style> --- class: left # The Problem with Diffusions .pull-left[ .bold.text-shadow.red[Random Walk Metropolis] <body><br> 1) Given the current state \(\q_n\), propose a new state $$ \q_{n+1} \sim \kappa(\q|\q_n) $$ 2) Accept / Reject with probability $$ \alpha(\q_{n+1} | \q_n) = 1 \wedge \f{\kappa(\q_{n}|\q_{n+1})\pi(\q_{n+1})}{\kappa(\q_{n+1}|\q_{n})\pi(\q_{n})} $$ </body>] -- .pull-right[ <img src=images/gifs/rw2.gif height="400"/> ] -- .content-box-orange.center[ Wastes too much time in high dimensions. ] --- class: left # More intelligent diffusions .pull-left[ .bold.text-shadow.red[Langevin Monte Carlo] <body><br> 1) Given the current state \(\q_n\), propose a new state $$ \q_{n+1} \sim \q_n + {h}\D\log\pi(\q_n) + 2h \N(\zerov, \Sigma) $$ 2) Accept / Reject with probability $$ \alpha(\q_{n+1} | \q_0) = 1 \wedge \f{\kappa(\q_{n}|\q_{n+1})\pi(\q_{n+1})}{\kappa(\q_{n+1}|\q_{n})\pi(\q_{n})} $$ </body>] -- .pull-right[ <img src=images/gifs/l2.gif width="400"/> ] -- .content-box-orange.center[ Only works for small step sizes! ] --- class: inverse, center, middle count: false # Hamiltonian Monte Carlo --- class: center, middle .center[ <img src=https://i.imgflip.com/6ci50a.jpg width=70%> ] --- class: left # Why walk when you can flow? 1. Augment state space with auxiliary variables `\(\p \sim \N(\boldsymbol{0}, \S)\)` 2. Joint distribution `\((\q,\p) \sim \exp(-H(\q,\p))\)` where `\(H(\q,\p)\)` is given by `\begin{align} H(\q,\p) = U(\q) + \f{1}{2} \p^{\top}\S^{-1}\p \end{align}` 3. Treat `\(H(\q,\p)\)` as the Hamiltonian of a system and generate trajectories using Hamiltonian dynamics <body>$$\dd{t}\begin{bmatrix}\q_t\\ \p_t\end{bmatrix} = \begin{bmatrix}\Ov & \I\\ -\I & \Ov\end{bmatrix}\begin{bmatrix}\D_{\!\q}H(\q_t, \p_t)\\ \D_{\!\p}H(\q_t, \p_t)\end{bmatrix} = \begin{bmatrix}\S\inv\p_t\\ - \D U(\q_t)\end{bmatrix}.$$ </body> 1. Accept/reject state `\((\q_t, \p_t)\)` with probability .center.content-box-blue[<body>$$\alpha(\q_t, \p_t | \q_0, \p_0) = \min\pb{1, \f{\kappa(\q_0, \p_0 | \q_t, \p_t) e^{-H(\q_t,\p_t)}}{\kappa(\q_t, \p_t | \q_0, \p_0) e^{-H(\q_0,\p_0)}} \Bigg| \ddd{(\q_0, \p_0)}{(\q_t, \p_t)} \Bigg| }.$$ </body>] --- class: center count: false # .left[Why walk when you can flow?] <img src=images/gifs/h1.gif height="500"/> --- class: center count: false # .left[Why walk when you can flow?] <img src=images/gifs/h2.gif height="500"/> --- class: center count: false # .left[Why walk when you can flow?] <img src=images/gifs/h3.gif height="500"/> --- class: center count: false # .left[Why walk when you can flow?] <img src=images/gifs/h4.gif height="500"/> --- # The Four Pillars of Hamiltonian Monte Carlo .panelset[ .panel[.panel-name[Energy Conservation] .pull-left[ <br><br> .theorem[<body> For every trajectory \(\pb{(\q_t, \p_t) : t > 0}\) satisfying the Hamiltonian dynamics $$ \dd{t}H(\q_t, \p_t) = 0. $$ </body>] ] .pull-right[ <img src=images/gifs/wo-friction2.gif height="270" /> ] <br> .content-box-blue.center[<body> \(H(\q_t, \p_t) = H(\q_0, \p_0)\) </body>] ] .panel[.panel-name[Reversibility] .pull-left[ <br> .theorem[<body>Let:<br><br> 1. \(\flip: (\q, \p) \mapsto (\q, -\p)\) denote a .bolder.text-shadow[momentum flip]<br> 2. \(\F_t: (\q_0, \p_0) \mapsto (\q_t, \p_t)\) be the .bolder.text-shadow[flow operator]<br><br> Then $$ \flip \circ \F_t \circ \flip \circ \F_t = \id. $$ </body>] ] .pull-right[ <img src=images/gifs/reversible.gif height="270"/> ] <br> .content-box-blue.center[<body> Detailed balance condition is satisfied, i.e., \(\kappa(\q_t, \p_t | \q_0, \p_0) = \mathbf{1}.\) </body>] ] .panel[.panel-name[Volume Preservation] .pull-left[ <br> .theorem[<body> For every trajectory \(\pb{(\q_t, \p_t) : t > 0}\)$$\ddd{(\q_0, \p_0)}{(\q_t, \p_t)} = \Bigg| \begin{bmatrix} \ddd{\q_0}{\q_t} & \ddd{\q_t}{\p_0} \\ \ddd{\p_t}{\q_0} & \ddd{\p_t}{\p_0} \end{bmatrix} \Bigg| = 1.$$ </body>] ] .pull-right[  ] <br> .content-box-blue.center[<body>$$\alpha(\q_t, \p_t | \q_0, \p_0) = \min\pb{1, \f{\kappa(\q_0, \p_0 | \q_t, \p_t) e^{-H(\q_t,\p_t)}}{\kappa(\q_t, \p_t | \q_0, \p_0) e^{-H(\q_0,\p_0)}} \Bigg| \ddd{(\q_0, \p_0)}{(\q_t, \p_t)} \Bigg| } = 1.$$ </body>] ] .panel[.panel-name[Symplecticity] .pull-left[ <br> .theorem[<body>Let \(\omega(\cdot, \cdot)\) be the .bolder.text-shadow[symplectic 2-form] $$ \omega(\q,\p) = \sum_{i=1}^d dq_i \wedge dp_i. $$ Then \(\omega(\q_t, \p_t) = \omega(\q_0, \p_0)\). </body>] ] .pull-right[ 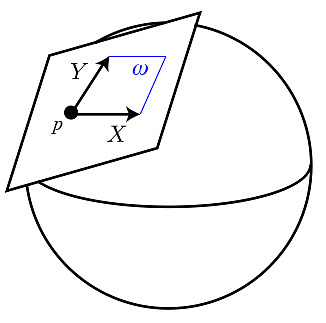 ] .content-box-blue.center[<body> Enables efficient numeric approximations. </body>] ] ] --- class: left # Symplectic Integration * The solution `\(\F_t: (\q_0, \p_0) \mapsto (\q_t, \p_t)\)` to the system of .bolder.text-shadow.dblue[differential equations] $$ \ddd{t}{\q_t} = \S\inv\p_t, \quad\quad \ddd{t}{\p_t} = - \D U(\q_t) $$ is rarely available in practice. It can be numerically solved using the .bolder.red.text-shadow[leapfrog scheme].<br> -- * Let `\(\e \approx dt\)` be a small .bolder.red[step-size], then `\(\F_{dt} \approx \F_\e: (\q_t, \p_t) \mapsto (\q_{t+\e}, \p_{t+\e})\)` is given by .content-box-red.center[<body>$$\begin{cases} \p_{t+\f \e 2} = \p_t - \f \e 2 \D U(\q_t) \\[5pt] \q_{t+\e} = \q_t + \e \S\inv \p_{t+\f \e 2}\\[5pt] \p_{t+\e} = \p_{t+\f \e 2} - \f \e 2 \D U(\q_{t+\e}) \end{cases} $$ </body>] -- <br> .content-box-orange.center[<body>For time \(T\) and \(L = \lfloor{T/\e}\rfloor\), we have \(\F_T \approx \F_{\e, L} = (\F_\e)^{\otimes L}\). </body>] --- class: center # .left[Why symplectic integration?] <img src=images/intro/symp2.png width="70%"> -- .content-box-orange[<body> `\(|H\left( \F_{T}(\q,\p) \right) - H\left( \F_{\e, L}(\q,\p) \right)| \approx O(\e^3)\)`. Therefore, `\(\alpha(\q_T, \p_T | \q_0, \p_0) \approx e^{O(\e^3)}.\)`</body>] --- class: left # .left[Is HMC the panacea?] .theorem-blue[<body>For \(b \in \R\) and \(\muv = b \mathbf{1}_d\) consider the multimodal target $$ \pi(\q) \sim \half \mathcal{N}(\q | -\muv, \I_d) + \half \mathcal{N}(\q | +\muv, \I_d), $$ and let \(\mathcal{E}(b, d)\) denote the event that for initial state \(\q_0=-\muv\) $$ \mathcal{E}(b, d) = \pb{ \norm{\P_T(\q_0) - \muv} \le \norm{\P_T(\q_0) + \muv}} $$ Then for all \(b > \sqrt{2/d}\) $$ \pr\pa{\mathcal{E}(b, d)} \le \exp\bigg(-\f{1}{8} \pa{\f{b^2d - 2}{b}}^2\bigg) $$ </body>] .center[<body>Markov chain mixes well only when `\(U(\q)\)` is strongly convex. Terrible for multimodal distributions.</body>] --- class: center count: false # .left[Is HMC the panacea?] <img src=images/gifs/hm1.gif height="400" width="400"/> Markov chain mixes well only when `\(U(\q)\)` is strongly convex. Terrible for multimodal distributions. --- class: center count: false # .left[Is HMC the panacea?] <img src=images/gifs/hm2.gif height="400" width="400"/> Markov chain mixes well only when `\(U(\q)\)` is strongly convex. Terrible for multimodal distributions. --- class: inverse, center, middle count: false # HaRAM ## Hamiltonian Repelling-Attracting Metropolis --- class: left # Observation #1: .orange.text-shadow[Friction dissipates energy] Consider the trajectory of a particle `\((\q_t, \p_t)\)` on a rough surface with friciton `\(\gamma > 0\)` $$ \ddd{t}{\q_t} = \S\inv\p_t, \quad\quad \ddd{t}{\p_t} = - \D U(\q_t) - \gamma\p_t $$ This can be rewritten as <body>$$\dd{t}\begin{bmatrix}\q_t\\ \p_t\end{bmatrix} = \underbrace{\begin{bmatrix}\Ov & \I\\ -\I & \Ov\end{bmatrix}}_{=\Om}\underbrace{\begin{bmatrix}\D_{\!\q}H(\q_t, \p_t)\\ \D_{\!\p}H(\q_t, \p_t)\end{bmatrix}}_{=\D H(\q_t, \p_t)} - \underbrace{\begin{bmatrix}\Ov & \Ov\\ \Ov & \gamma\I\end{bmatrix}}_{=\G}\begin{bmatrix}\q_t\\ \p_t\end{bmatrix}.$$ </body> -- Therefore, <body>$$\dd{t}(\q_t, \p_t) = \Om \D H(\q_t, \p_t) - \G (\q_t, \p_t).\tag{A}$$ </body> -- <br> .content-box-blue.center[<body>Eq. (A) is called a .text-shadow.red[conformal Hamiltonian system]. </body>] --- class: left count: false # Observation #1: .orange.text-shadow[Friction dissipates energy] .center[ <img src=images/gifs/wo-friction.gif height="400"/> ] --- class: left count: false # Observation #1: .orange.text-shadow[Friction dissipates energy] .center[ <img src=images/gifs/w-friction.gif height="400"/> ] --- class: left # Observation #2: .orange.text-shadow[Negative friction accumulates energy] If we just flip the sign of the friction parameter `\(\gamma\)` we get -- <br><br> <body>$$\dd{t}\begin{bmatrix}\q_t\\ \p_t\end{bmatrix} = \underbrace{\begin{bmatrix}\Ov & \I\\ -\I & \Ov\end{bmatrix}}_{=\Om}\underbrace{\begin{bmatrix}\D_{\!\q}H(\q_t, \p_t)\\ \D_{\!\p}H(\q_t, \p_t)\end{bmatrix}}_{=\D H(\q_t, \p_t)} + \underbrace{\begin{bmatrix}\Ov & \Ov\\ \Ov & \gamma\I\end{bmatrix}}_{=\G}\begin{bmatrix}\q_t\\ \p_t\end{bmatrix}.$$ </body> i.e., <body>$$\dd{t}(\q_t, \p_t) = \Om \D H(\q_t, \p_t) + \G (\q_t, \p_t).\tag{B}$$ </body> -- <br> .content-box-blue.center[<body> Eq. (B) is also a .text-shadow.red[conformal Hamiltonian system.] </body>] --- class: left count: false # Observation #1: .orange.text-shadow[Negative friction accumulates energy] .center[ <img src=images/gifs/wo-friction2.gif height="400"/> ] --- class: left count: false # Observation #1: .orange.text-shadow[Negative friction accumulates energy] .center[ <img src=images/gifs/neg-friction.gif height="400"/> ] .content-box-blue.center[<body>But as `\(t \uparrow\)`, the particle magically gains energy (हराम) </body>] --- class: center, middle count: false # HaRAM ## / हराम / .Large[Proscribed or forbidden by Law.] --- # Hamiltonian Repelling-Attracting Metropolis 1. Choose a hypothetical friction parameter `\(\gamma \in [0, \infty)\)`, and integration time `\(T\)` 2. Let `\(\Om = \begin{bmatrix}\boldsymbol{0} & I\\ -I & \boldsymbol{0}\end{bmatrix}\)` and `\(\G = \begin{bmatrix} \boldsymbol{0} & \boldsymbol{0} \\ \boldsymbol{0} & \gamma \end{bmatrix}\)` 3. For time `\(t \in [0, T/2]\)` generate .dblue[conformal Hamiltonian dynamics] using .red[negative friction] `\begin{align} \f{d}{d t} (\q_t, \p_t) = \Om \D H(\q_t, \p_t) + \G (\q_t, \p_t) \end{align}` -- 1. For time `\(t \in [T/2, T]\)` generate .dblue[conformal Hamiltonian dynamics] using .green[positive friction] `\begin{align} \f{d}{d t} (\q_t, \p_t) = \Om \D H(\q_t, \p_t) - \G (\q_t, \p_t) \end{align}` -- 1. Accept/reject state `\((\q_T, \p_T)\)` with MH probability <body>$$\alpha(\q_T, \p_T | \q_0, \p_0) = \min\pb{1, \f{\kappa(\q_0, \p_0 | \q_T, \p_T) e^{-H(\q_T,\p_T)}}{\kappa(\q_T, \p_T | \q_0, \p_0) e^{-H(\q_0,\p_0)}} \Bigg| \ddd{(\q_0, \p_0)}{(\q_t, \p_t)} \Bigg| }$$ </body> --- count: false # Hamiltonian Repelling Attracting Metropolis .center[ <img src=images/gifs/downup.gif height="400"/> ] --- count: false # Hamiltonian Repelling Attracting Metropolis .center[ <img src=images/gifs/downup2.gif height="400"/> ] --- count: false # Hamiltonian Repelling Attracting Metropolis .center[ <img src=images/gifs/downup3.gif height="400"/> ] --- class: left # Properties of HaRAM .panelset[ .panel[.panel-name[Energy] .left.content-box-purple[<body> For the Haram trajectory \(\pb{(\q_t, \p_t) : t > 0}\)$$\dd{t}H(\q_t, \p_t) \neq 0,$$but$$\int\limits_0^T\dd{t}H(\q_t, \p_t) dt = 0.$$ </body>] <br> .content-box-green.center[<body> This implies that \(H(\q_t, \p_t) = H(\q_0, \p_0)\) only when \(t=T\).</body>] ] .panel[.panel-name[Reversibility] .pull-left[ .left.content-box-purple[<body> Let .red[<body>\(\F_t\)</body>] and .green[<body>\(\P_t\)</body>] be the conformal .bolder.text-shadow[Hamiltonian flow operators] w.r.t. the .red[repelling] and .green[attracting] dynamics, and let \(\flip\) be the .bold[momentum-flip] operator. Then for all \(t \le T/2\)$$\flip \circ \P_t \circ \flip = \F_{-t}$$ In particular,$$\flip \circ \pa{\P_T \circ \F_T} \circ \flip \circ \pa{\P_T \circ \F_T} = \id.$$ </body>] <br> .content-box-green.center[<body> This implies that \(\kappa(\q_T, \p_T | \q_0, \p_0) = \onev\).</body>] ] .pull-right[ <img src="images/gifs/reversible.gif" height="270"/> ] ] .panel[.panel-name[Volume] .left.content-box-purple[<body> .red[<body>\(\F_t\) expands volume</body>] and .green[<body>\(\P_t\) shrinks volume</body>]. But, over the entire time interval \([0,T]\):$$\ddd{(\q_0, \p_0)}{(\q_T, \p_T)} = 1.$$ </body>] <br> .content-box-green.center[<body> This implies that the Jacobian correction term \(\Bigg|\ddd{(\q_0, \p_0)}{(\q_T, \p_T)}\Bigg|\) is not needed.</body>] ] .panel[.panel-name[Symplecticity] .left.content-box-purple[<body>The deformation of the .bolder.text-shadow[symplectic 2-form] is given by $$ \omega(\q_t, \p_t) = \begin{cases} e^{\gamma t}\omega(\q_0, \p_0), & 0 \le t \le T/2\\ \\ e^{\gamma (T-t)}\omega(\q_0, \p_0), & T/2 \le t \le T \end{cases}. $$ In particular, HaRAM preserves the .bolder.text-shadow[symplectic 2-form], i.e.,$$\omega(\q_T, \p_T) = \omega(\q_0, \p_0).$$</body>] ] .panel[.panel-name[Numerical Integration] For `\(\e \approx dt\)` and the .red[repelling] conformal Hamiltonian flow `\(\F_\e \approx \F_{dt}\)` the .red[conformal symplectic leapfrog algorithm] is $$ `\begin{cases} \tp_{t+\f \e 2} = e^{\gamma\e/2}\p_t \\[5pt] \p_{t+\f \e 2} = \tp_{t+\f \e 2} - \f \e 2 \D U(\q_t) \\[5pt] \q_{t+\e} = \q_t + \e\S\inv \tp_{t+\f \e 2}\\[5pt] \tp_{t+\e} = \tp_{t+\f \e 2} - \f \e 2 \D U(\q_{t+\e})\\[5pt] \p_{t+\e} = e^{\gamma\e/2}\p_{t+\e} \end{cases}` $$ and for `\(L = \lfloor T/\e \rfloor\)`, `\(\F_T \approx \F_{\e, L} = (\F_\e)^{\otimes L}\)`. Similarly, `\(\P_T \approx \P_{\e, L} = (\P_\e)^{\otimes L}\)` by changing the sign of `\(\gamma\)`. .content-box-purple.center[<body> `\(|H\left( \P_{T} \circ \F_{T}(\q,\p) \right) - H\left( \P_{\e, L} \circ \F_{\e, L}(\q,\p) \right)| \approx O(\e^3)\)`</body>] ] ] --- class: inverse, center, middle count: false # Experiments --- class: left # Bimodal Gaussian .panelset[ .panel[.panel-name[Setup] .pull-left[ For `\(\muv = 5 \onev_d \in \R^d\)` the target is $$ \pi(\q) \sim \half \mathcal{N}(\muv, \Sigma_1) + \half \mathcal{N}(-\muv, \Sigma_2) $$ where * `\(\Sigma_1(i, j) = 0.5^{|i-j|}\)` <br> * `\(\Sigma_2 = R \Sigma_1 R^\top\)` for `\(R \in SO(d)\)` <br> .content-box-blue[<body> `\(d \in \pb{2, 10, 50}\)` for HaRAM, HMC, RAM & PEHMC</body>] ] .pull-right.center[ <img src="images/plots/gaussian3/d/plt.svg"> ] .panel[.panel-name[Results] | Method | Time/L(d=2) | Time/L(d=10) | Time/L(d=50) | W2 metric (d=2) | W2 metric (d=10) | W2 metric (d=50) | |-------:|:-----------:|:------------:|:------------:|:---------------:|:----------------:|:----------------:| | HaRAM | 0.2697 s | 0.7125 s | 2.9816 s | 0.51 | 7.93 | 7.78 | | HMC | 0.2555 s | 0.6602 s | 2.9375 s | 17.16 | 45.93 | 48.04 | | RAM | 0.5364 s | 0.5847 s | 33.7778 s | 0.9 | 33.88 | 51.52 | | MHMC | 0.5211 s | 1.3530 s | --- | 18.41 | 89.50 | 47.84 | ] .panel[.panel-name[Mixing] .pull-left[.center[ <img src="images/plots/gaussian3/d/plt2_acf.svg"> d=2 ]] .pull-right[.center[ <img src="images/plots/gaussian3/d/plt10_acf.svg"> d=10 ]] ] .panel[.panel-name[Trace] .pull-left.center[ <img src="images/plots/gaussian3/d/plt2_tr.svg"> ] .pull-right.center[ <img src="images/plots/gaussian3/d/plt10_tr.svg"> ] ] .panel[.panel-name[Scatter] .pull-left.center[ <img src="images/plots/gaussian3/d/scatter2.svg"> d=2 ] .pull-right.center[ <img src="images/plots/gaussian3/d/scatter10.svg"> d=10 ] ] .panel[.panel-name[Parallel Tempering (d=2)] <img src="images/plots/gaussian3/d/plt2_tr_all.svg" height="60%"> ] .panel[.panel-name[Parallel Tempering (d=10)] <img src="images/plots/gaussian3/d/plt10_tr_all.svg" height="60%"> ] ] ] --- class: left # Benchmark Dataset .panelset[ .panel[.panel-name[Setup] .center[ <img src="figures/sims/gaussian3/d2.svg"> .content-box-blue[<body> `\(20\)` component Gaussian Mixture given in Kou et al. (2006)</body>] ] ] .panel[.panel-name[Mixing] .pull-left.center[ <br><br> | Method | Time/L | W2 metric | |-------:|:-----------:|:---------------:| | HaRAM | 0.2697 s | 133.733 | | HMC | 0.2555 s | 2989.539 | | RAM | 0.5364 s | 309.421 | | MHMC | 0.5211 s | 1916.740 | ] .pull-right.center[ <img src="figures/sims/gaussian3/acf-d2.svg"> ] ] .panel[.panel-name[Trace] .center[ <img src="figures/sims/gaussian3/trace-d2.svg"> ] ] .panel[.panel-name[Scatter] .center[ <img src="figures/sims/gaussian3/scatter-d2.svg"> ] ] ] --- class: left # Vanilla Gaussian Distribution .pull-left.center[ <img src="figures/sims/gaussian1/d2.svg"> ] -- .pull-right.center[ <img src="figures/sims/gaussian1/acf-d2.svg"> ] --- class: left # Autotuning .panelset[ .panel[.panel-name[Setup] For `\(\muv = \frac{5}{\sqrt{d}} \onev_d \in \R^d\)` the target is $$ \pi(\q) \sim \half \mathcal{N}(\muv, I) + \half \mathcal{N}(-\muv, I) $$ .content-box-blue[<body> Nesterov dual averaging for `\((\epsilon, \gamma, L)\)` </body>] .panel[.panel-name[d=3] .center[ <img src="images/plots/autotune/d3.svg" height="350"> ] ] .panel[.panel-name[d=10] .center[ <img src="images/plots/autotune/d10.svg" height="350"> ] ] .panel[.panel-name[d=50] .center[ <img src="images/plots/autotune/d50.svg" height="350"> ] ] .panel[.panel-name[d=100] .center[ <img src="images/plots/autotune/d3.svg" height="350"> ] ] ] ] --- class: left # Bayesian Neural Network .panelset[ .panel[.panel-name[Setup] .pull-left[ <img src="images/plots/nn/scatter.svg"> ] .pull-right.center[ <img src="images/plots/nn/nn.png"> ] .panel[.panel-name[Posterior prediction surface] <img src="images/plots/nn/contourf.svg"> ] .panel[.panel-name[Posterior prediction quantiles] .center[ <img src="images/plots/nn/all.svg"> ] ] ] ] --- class: left # Time Delay Estimation .panelset[ .panel[.panel-name[Gravitational Lensing] .pull-left[ <img src="images/plots/astro/lensing.jpg"> ] .pull-right[ <img src="images/plots/astro/ts-original.svg" height="250"> $$ `\begin{align} dX(t)&=-\frac{1}{\tau}(X(t)-\mu)dt+\sigma dB(t)\\ Y(t)&=X(t-\Delta)+\beta \end{align}` $$ ] .panel[.panel-name[Model] .pull-left[ $$ `\begin{align} x_i\mid X(t_i) &\sim N(X(t_i),~ \sigma^2_{x, i})\\ y_i\mid Y(t_i) &\sim N(Y(t_i),~ \sigma^2_{y, i})~~\textrm{and}\\ y_i\mid X(t_i-\Delta), \Delta, \beta &\sim N(X(t_i-\Delta) + \beta,~ \sigma^2_{y, i}), \end{align}` $$ $$ `\begin{align} \Delta &\sim \textrm{Unif}(-1178.939,~ 1179.939)\\ \beta &\sim \textrm{Unif}(-60,~ 60)\\ \mu &\sim \textrm{Unif}(-30,~ 30)\\ \sigma^2 &\sim \textrm{inverse-Gamma}(1, 2\times 10^{-7})\\ \tau &\sim \textrm{inverse-Gamma}(1, 1). \end{align}` $$ ] .pull-right[<img src="images/plots/astro/hist.svg">] ] .panel[.panel-name[Aligned Light Curves] .center[ <img src="images/plots/astro/match.svg"> ] ] ] ] --- class: left # Code .pull-left[ ```julia using main using Distributions, DynamicPPL, Plots x = randn(1000) y = x .+ randn(1000) .* e @model function lr(x, y) σ2 ~ Truncated(Normal(), 1e-6, Inf) b0 ~ Cauchy(2.0) b1 ~ Normal(0.0, 1.0) y ~ MvNormal(b0 .+ b1 .* x, σ2 * I) end samples, accepts = mcmc( DualAverage(λ=10, δ=0.8) HaRAM(); lr(x, y), n=1e4, n_burn=1e4 ); ``` ] .pull-right[ <img src="images/plots/qr.png"> .content-box-blue.center[<body> github.com/sidv23/haram </body>] ] ``` --- # References 1. Arnold, V. I. (2013). *Mathematical methods of classical mechanics* 2. Betancourt, M. (2017). *A Conceptual Introduction to Hamiltonian Monte Carlo* 3. Betancourt, M., Byrne, S., Livingstone, S. & Girolami, M. (2017). *The geometric foundations of Hamiltonian monte carlo* 4. Duane, S., Kennedy, A. D., Pendleton, B. J. & Roweth, D. (1987). *Hybrid Monte Carlo* 5. Hairer, E., Lubich, C. & Wanner, G. (2006). *Geometric Numerical Integration* 6. McLachlan, R. & Perlmutter, M. (2001). *Conformal Hamiltonian systems* 7. Neal, R. (2011). *MCMC Using Hamiltonian Dynamics* 8. Tak, H., Meng, X.-L. & van Dyk, D. A. (2018). *A repelling-attracting Metropolis algorithm for multimodality.* 9. Tierney, L. (1994). *Markov chains for exploring posterior distributions.* 10. Tripuraneni, N., Rowland, M., Ghahramani, Z. & Turner, R. (2017). *Magnetic Hamiltonian Monte Carlo* --- class: inverse, center, middle # Thanks for listening! ## Questions?